The emergence of large language models (LLMs) has sparked significant interest and excitement across various fields, including life sciences. These powerful computational tools, fueled by vast amounts of data and advanced machine learning techniques, have the potential to transform our approach towards healthcare and medical research. However, it is also essential to recognize that LLMs in clinical research are not without their limitations and challenges.
In this blog, we will explore the challenges surrounding the integration of LLMs in clinical trials. From issues related to data quality and bias to ethical considerations and the practical challenges of implementation, we shall try and understand the nuanced landscape of LLMs in clinical research.
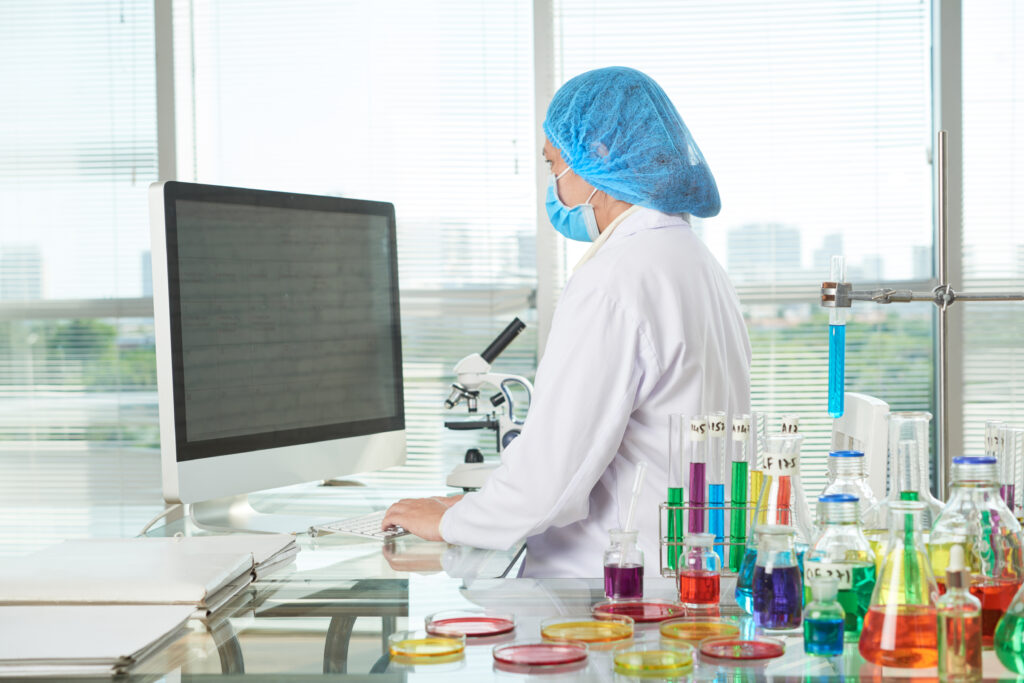
What are the major challenges of LLMs in clinical research?
a). Data Quality & Bias
b). Interpretability & Transparency
c). Ethical Considerations
d). Generalization & Robustness
e). Resource Intensiveness
Now let us understand the challenges in detail.
– Data Quality & Bias:
It is an undeniable fact that the quality of the data driving the analysis is the cornerstone of effective clinical research. Among the top challenges of LLMs in clinical research are the data quality and biases. Clinical datasets are diverse, reflecting various demographics, healthcare practices, and geographical regions. Yet, within this diversity lies the potential for biases, whether due to underrepresentation of certain populations or systematic errors in data collection.
These biases can influence the outputs generated by LLMs, leading to inaccurate results. For instance, if a dataset predominantly includes patient data from urban areas, LLMs trained on this data may not adequately capture the healthcare needs and outcomes of rural communities. Similarly, biases related to age, gender, ethnicity, or socioeconomic status can impact the generalizability and fairness of LLM-based predictions and recommendations.
– Interpretability & Transparency:
Transparency and interpretability are also major challenges of LLMs in clinical research. Researchers rely on understanding the reasoning behind computational models’ predictions to make informed decisions about patient care and treatment strategies. However, large language models often operate as opaque “black boxes”, complicating efforts to interpret their outputs and understand the factors driving their recommendations.
The lack of interpretability in LLMs stems from their complex architectures and the sheer volume of parameters involved in their training. Unlike traditional statistical models which provide explicit rules or coefficients to explain their predictions, LLMs generate outputs based on intricate patterns learned from vast amounts of data. Deciphering how these models arrive at their conclusions can be challenging, if not impossible, without additional tools and methodologies.
Clinical professionals may be hesitant to trust or act upon LLM-generated insights if they cannot understand the rationale behind them. Moreover, regulatory bodies and ethics committees may require transparent explanations of how LLMs make decisions to ensure patient safety and data privacy.
Ethical Considerations:
Ethical considerations is one of the biggest challenges of LLMs in clinical research. The advent of large language models gave rise to new ethical questions, adding layers of complexity to an already complicated landscape. Patient privacy and consent is a pressing ethical concern surrounding LLMs. Clinical research relies on access to vast amounts of sensitive patient data, including medical records, genetic information, and diagnostic imaging. Training LLMs on such data raises questions about informed consent and data protection.
Ensuring that patients understand and consent to the use of their data for model development is essential for upholding ethical standards and respecting individuals’ autonomy. Furthermore, LLMs have the potential to perpetuate or exacerbate existing healthcare disparities if not carefully calibrated and validated across diverse populations. Biases present in the training data can lead to disparities in model predictions, affecting the quality and fairness of healthcare delivery.
– Generalization & Robustness:
Ensuring the reliability and robustness of LLMs in clinical research is a critical challenge facing clinical professionals. While LLMs have demonstrated impressive performance on benchmark tasks, their ability to generalize to diverse patient populations and robustness to input variations remain areas of concern. Clinical research deals with complex and heterogeneous data, encompassing a wide range of medical conditions, treatment modalities, and patient demographics.
LLMs trained on specific datasets may struggle to generalize their learnings to unseen scenarios, leading to suboptimal performance or even errors in predictions. Moreover, variations in data quality, such as missing data, can further challenge the robustness of LLMs in clinical research. Addressing the generalization and robustness challenges of LLMs requires rigorous evaluation and validation across diverse datasets and clinical scenarios.
– Resource Intensiveness:
While the integration of LLMs in clinical research offer promising potential, their practical implementation is hindered by significant resource requirements. Training and fine-tuning LLMs demand substantial computational power, expertise, and data infrastructure, especially those in resource-limited settings. The computational resources needed to train LLMs can be prohibitive, requiring high-performance computing clusters and specialized hardware accelerators.
Additionally, the expertise required to navigate the complexities of LLM development and deployment, including data preprocessing, model tuning, and performance evaluation, may not be readily available to all researchers. Furthermore, the data infrastructure necessary to support LLM-based research is often lacking in many clinical settings. Clinical datasets are typically dispersed across various healthcare systems and institutions, presenting challenges for data aggregation, standardization, and sharing.
Building robust data pipelines and collaborative networks to support LLM research requires significant investments in infrastructure and coordination efforts. Addressing the resource intensiveness of LLMs in clinical trials calls for innovative approaches to democratize access and facilitate collaboration.
In conclusion, the integration of LLMs in clinical research presents both opportunities and challenges. While LLMs hold promise for enhancing our understanding of clinical data and improving patient care, they are not without limitations. The journey of integrating LLMs in clinical research is fraught with complexities. However, by acknowledging these challenges and working collaboratively to address them, we can harness the potential of LLMs to advance medical knowledge and improve patient outcomes responsibly.
At Inductive Quotient Analytics (IQA), we understood the impact of GenAI and LLMs in clinical research early on. With site selection being an essential aspect of studies, we have decided to make the process seamless and simple with Site Insights. Our homegrown site selection platform helps sponsors pick a site for their trials in just a few clicks! Want to know more? Get in touch for a demo: hello@inductivequotient.com